How Generative UI is impacting online shopping experiences
May 25, 2024
In eCommerce, generative AI offers new possibilities for creating online storefronts. While AI chat bots and entirely AI-generated storefronts present challenges, the most promising approach lies in using AI to efficiently create and optimize context-specific user experiences.
In eCommerce, many online storefronts look remarkably similar due to standardization from platforms like Shopify which provide pre-designed templates and themes that many businesses us. Even with the flexibility offered by composable and headless trends, user experiences and interfaces often fail to differentiate based on user intent and context. Generative AI is put forward as a game-changer in online retail, potentially revolutionizing the industry. From our work with leading online brands, we observe three paths for generative AI in online storefronts: the primitive, the lunatic, and the middle ground.
The Primitive: AI Chat Bots
AI chat bots are the most obvious and common use case for eCommerce frontends. These bots, inspired by OpenAI's prompting UX, recommend products based on user inputs. For example, Zalando uses AI chat bots to suggest outfits. While useful, this approach feels like rushing to implement shiny technology rather than offering a superior user experience compared to today's content-driven, funnel-based interfaces. AI chat bots introduce additional UX frictions since users have to verbally formulate their context and needs, eventually raising the question: What problem does the command center user interface solve that a more sophisticated search bar cannot?
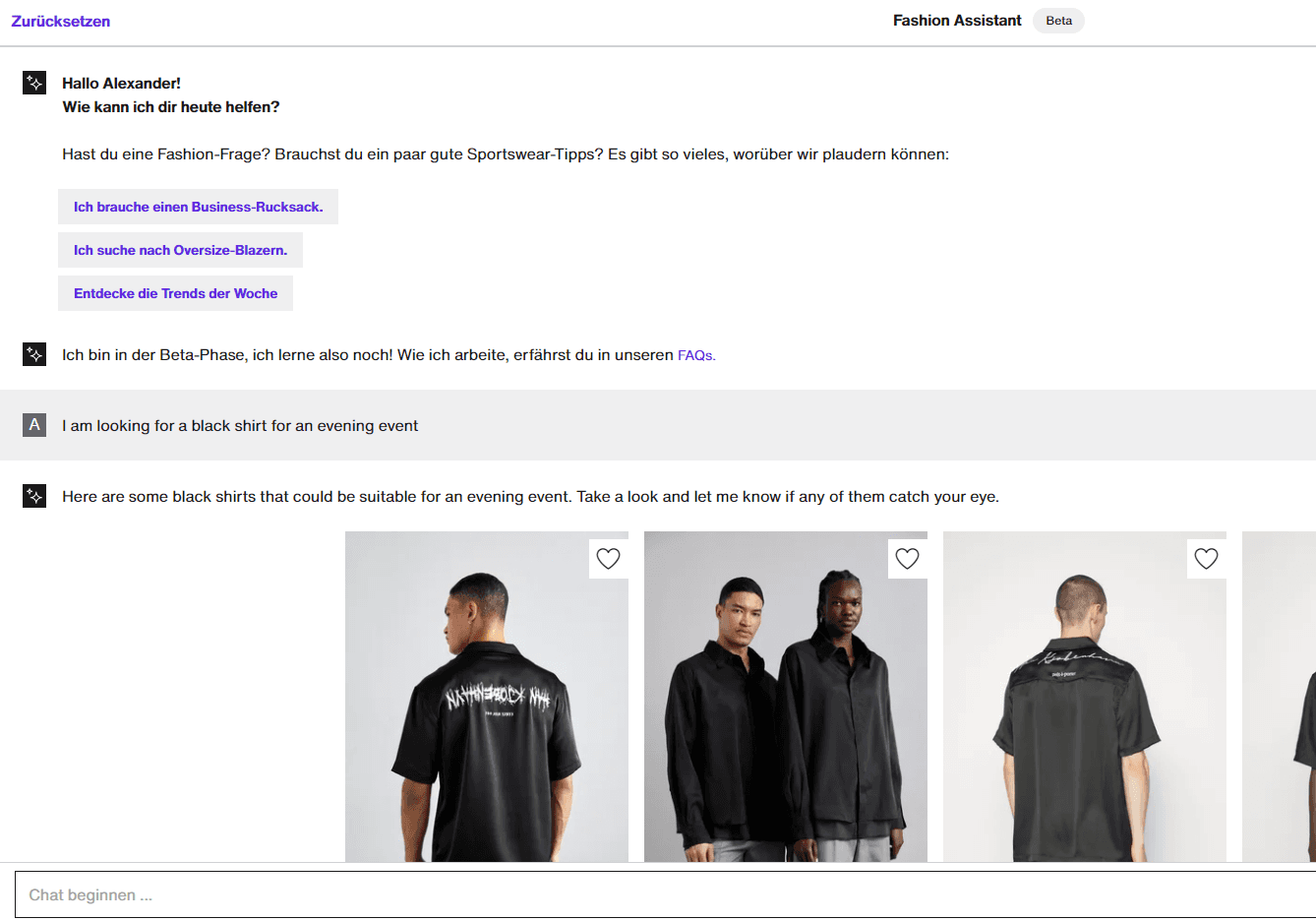
The Lunatic: Entirely AI-Generated Storefronts
On the more extreme end, some envision using generative AI to create entire storefronts based on user data and session behavior.
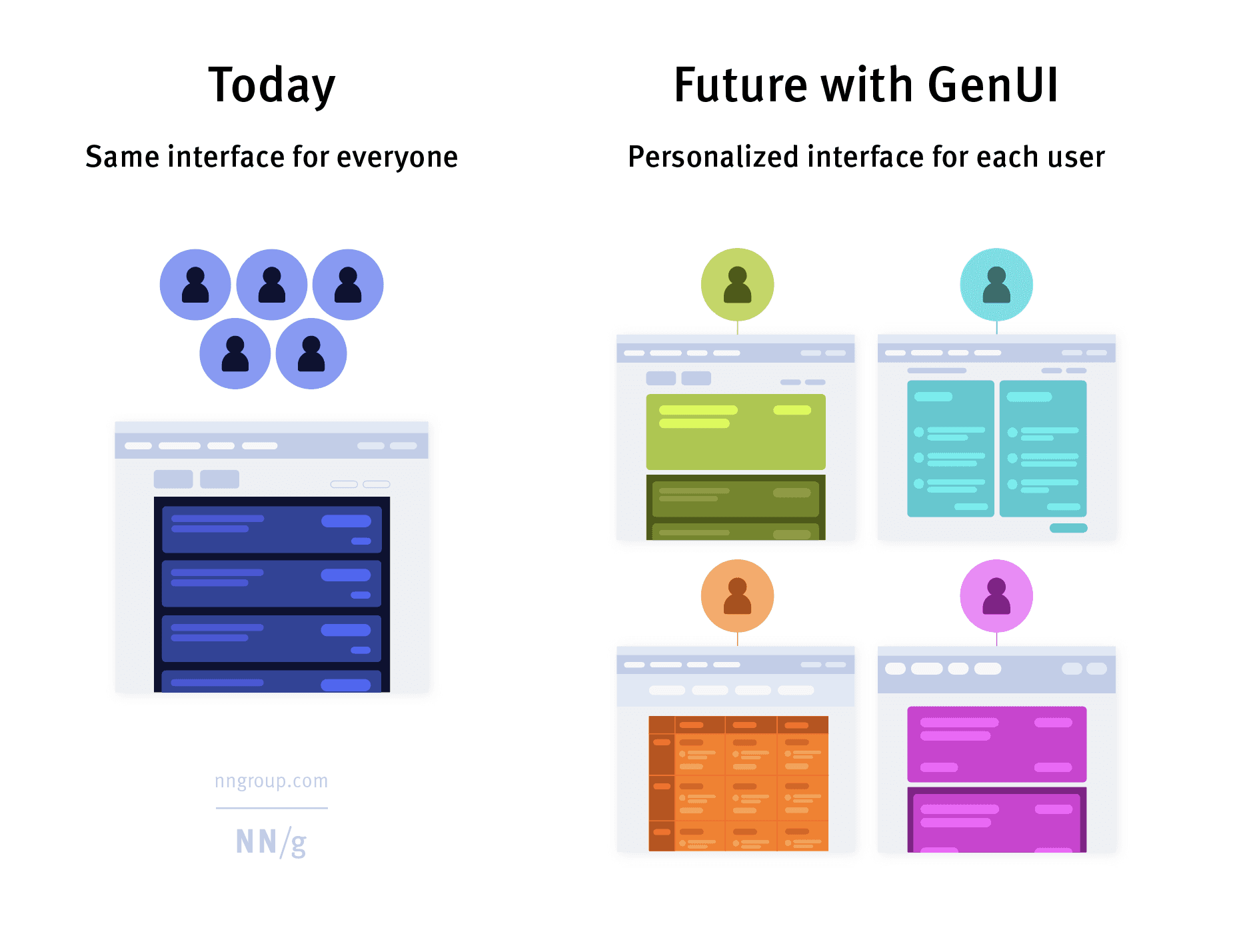
Generative UI or providing a truly personalized experience for every end user, generated "on the fly," sounds like an ambitious future goal. However, this future faces significant technical challenges, including latency, costs, and output quality. While these challenges will eventually be resolved, a more pressing issue is the need for rich user data to generate personalized experiences. With increasing restrictions on third-party data ("cookieless"), achieving true 1:1 personalization might not be feasible as long as we value privacy. Despite marketing tech vendors advocating for this level of personalization for over a decade, the primary bottleneck has always been limited user data and the complexity of implementing such solutions, particularly for smaller merchants. These limitations apply to AI-generated storefronts as well.
The Middle Ground: Context-Specific Experiences
The most promising approach lies in using software with “AI native workflows” to create context-specific experiences. Users can be clustered around different contexts: browsing, searching, short-bite high-paced video content, long-text informational content, familiar with the brand, or new visitors.
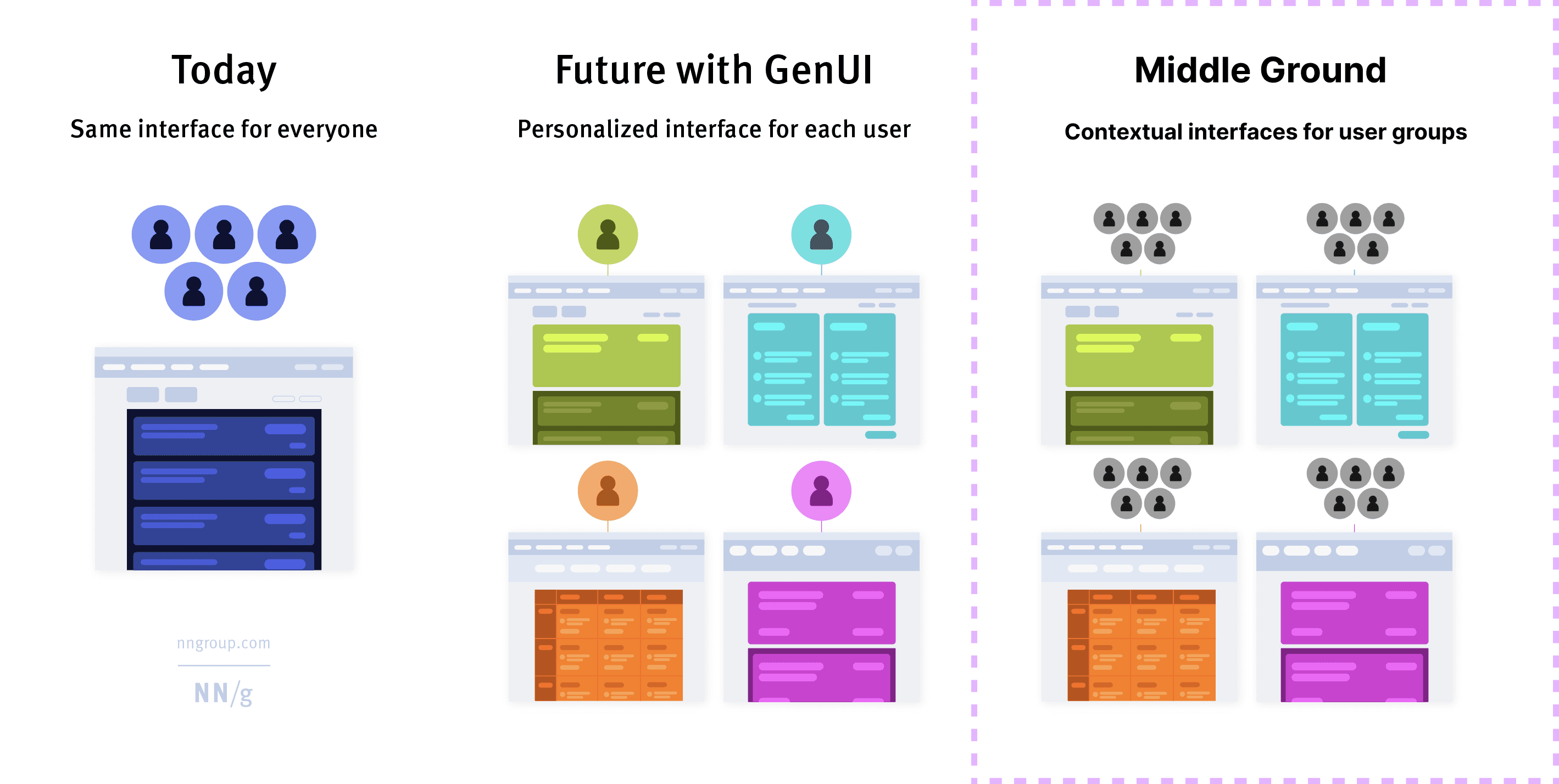
Different contexts require different interfaces, but a web experience targeted for a specific context is still too difficult today. Limitations in user data also apply here, as online merchants often lack knowledge about user intent and preferences. However, marketers can understand traffic sources and other key variables to determine how an experience should look for a specific context. For example, a visitor from a TikTok video ad might require a different storefront than a high-intent user searching for "best fitness leggings S" on Google.
Generative AI's greatest potential lies in equipping marketers with AI native workflow tools that make it easier to create contextual experiences without coding or design skills. Page builders with AI features already start solve the "blank page" problem, suggesting or creating the right layout for experiences based on few inputs. Generative UI tools like v0.dev can already be used to generate and iterate website components. Generate and edit text, image or video content to enrich experiences can also be done more efficiently as well as creating variants of single experiences. Tools like coframe already allow the implementation of dynamically serving AI generated variants of images or text assets, based on live data to optimize website performance. Next to variant creation, marketers can already leverage machine learning to automatically route traffic to the best experience based on set optimization goals.
While context specific storefronts can be created without AI today, it takes days or weeks to create high-converting experiences and require a complex lingo between designers, developers and marketers. AI will make the process more efficient and lead to more effective results. Rather than striving to magically generate storefronts, the more promising approach is to enable marketers to blend their intuition with AI native workflows and no-code tools. Marketing professionals know their products and customers best, and we should equip them with tools to create and test tailored, contextual experiences. Blending no-code capabilities with AI tools will unlock significant potential in online commerce.
We've seen firsthand the impact of this method, and as infrastructure, data, and tools improve, its effectiveness will only grow. Reach out if you want to discuss the future of commerce storefronts.